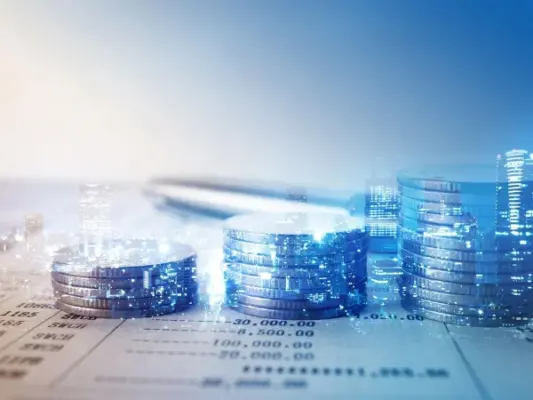
In the vast and dynamic business landscape , where traditional banking services coexist with cutting-edge digital innovations, the financial sector faces unique challenges. The continent’s rich tapestry of cultures, languages, and economic conditions has led to a disparity in access to financial services, with many rural regions lacking basic banking facilities. This gap has left a significant portion of the population unbanked, unable to access fundamental financial services. However, the mobile revolution has been a game-changer, enabling millions to access financial services via mobile phones, bypassing traditional banking infrastructure. ‘s leadership in mobile money accounts is a testament to its rapid adaptation to digital solutions, making it a global frontrunner in this space.
The Economic Landscape and Demographic Dynamics
The financial landscape is a complex mix of traditional banking services and digital innovations, reflecting the continent’s vast array of cultures, languages, and economic conditions. From bustling cities to rural heartlands, access to financial services varies greatly. This disparity has left a substantial portion of the population unbanked, without access to fundamental financial services. The mobile revolution has been instrumental in bridging this gap, enabling millions to access financial services via mobile phones, bypassing traditional banking infrastructure.
Key Challenges in the Financial Sector
Despite advancements in mobile banking, the financial sector faces several significant challenges. Traditional credit scoring models, while effective in many contexts, have left a significant portion of the population underserved, particularly in regions where access to banking is limited. The challenges posed by changing financial dynamics, the gig economy, digital banking, and more have necessitated a reevaluation of how we assess creditworthiness. In , where limited access to banking is a prominent issue, the adoption of alternative credit scoring methods driven by Artificial Intelligence (AI) holds great promise.
Unlocking Financial Inclusion Through AI and Alternative Data
AI-driven alternative credit scoring models have the potential to revolutionize the way financial institutions assess creditworthiness, particularly in regions like where access to traditional banking services is limited. By tapping into non-traditional data sources, machine learning algorithms, and collaborations with service providers, these models can bridge the financial inclusion gap. Moreover, understanding credit risk more accurately opens doors to tailored loan rates and terms, increasing the number of loans disbursed and identifying new customer markets. This not only benefits borrowers but also contributes to the growth of the financial sector .
Leveraging Alternative Credit Scoring for Financial Inclusion
In the context of ’s limited access to banking services, alternative credit scoring models powered by AI offer a lifeline to millions. These models can bridge the financial inclusion gap by leveraging non-traditional data sources, machine learning algorithms, and collaborations with service providers. This approach not only benefits borrowers by providing them with better access to credit but also contributes to the growth of the financial sector .
Addressing the Financial Literacy Gap
There is a notable deficiency in financial literacy across the continent. This gap affects the population’s ability to make informed financial decisions and effectively use available financial services. To address these challenges, banks and other industry players could reconsider their channel strategies, integrating digital and human experiences, and enhancing their B2C offerings by digitizing end-to-end processes for operational efficiency. This could result in significant cost reductions across various functions and improve customer-facing sales and care.
Opportunities and Challenges of Using AI and Alternative Data
Alternative data, such as mobile and social footprints, may enhance traditional credit scoring models and improve financial inclusion. A study in India found that machine learning models using mobile and social footprint variables can predict loan defaults more effectively than models that only use credit scores. This suggests that using alternative data can improve credit access for individuals without a credit score who would have been rejected by the traditional credit screening model. However, challenges remain, particularly in data integration and transfer, which may affect the performance of ICS, highly dependent on the quality and quantity of data.
Wrapping Up
AI-driven alternative credit scoring models have the potential to revolutionize the way financial institutions assess creditworthiness, particularly in regions like where access to traditional banking services is limited. Embracing these innovative approaches can empower individuals and businesses by providing them with better access to credit, ultimately fostering economic growth and financial stability across the continent. As we continue to explore the potential of AI-driven alternative credit scoring models to unlock financial inclusion , it’s clear that the future of financial services in the continent is bright, with AI and alternative data playing pivotal roles in shaping its trajectory.
Expanding the Discussion
To further enrich our understanding of the role of AI and alternative data in amplifying financial development , let’s delve deeper into the specifics of these technologies and their implications for the continent’s financial landscape.
The Role of AI in Credit Scoring
AI algorithms can analyze vast amounts of data to identify patterns and trends that traditional credit scoring models might miss. By incorporating alternative data sources, such as mobile transaction records, social media activity, and even satellite imagery, AI can provide a more comprehensive view of an individual’s financial behavior. This can lead to more accurate credit assessments and fairer lending practices, especially in regions where access to traditional banking services is limited.
Challenges in Implementing AI and Alternative Data
Despite the potential benefits, there are several challenges to implementing AI and alternative data in the n financial sector. These include data privacy concerns, the need for robust data infrastructure, and the challenge of integrating alternative data sources into existing credit scoring models. Addressing these challenges will require collaboration between financial institutions, technology providers, and regulatory bodies.
The Future of Financial Inclusion in
The adoption of AI and alternative data represents a significant step forward in the quest for financial inclusion in . By leveraging these technologies, financial institutions can reach unbanked populations, providing them with access to credit and financial services. This not only benefits individuals and businesses but also contributes to economic growth and development across the continent.
Conclusion
As we look to the future, it’s clear that AI and alternative data will play a crucial role in amplifying financial development . By overcoming the challenges and leveraging the opportunities presented by these technologies, we can work towards a more inclusive and prosperous financial sector. The journey ahead will require innovation, collaboration, and a commitment to using technology to bridge the financial inclusion gap.