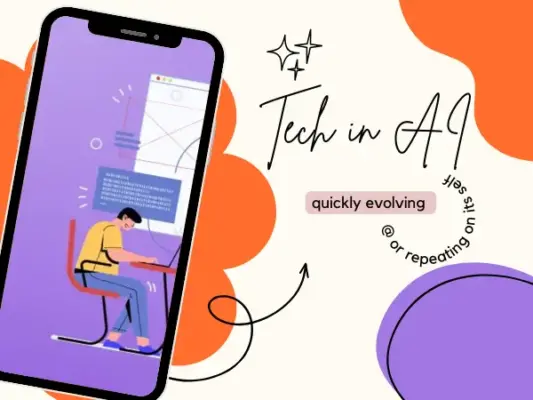
The field of artificial intelligence (AI) is often celebrated for its rapid advancements and revolutionary potential. However, some critics argue that, despite the hype, AI technology sometimes seems to recycle old concepts or show incremental improvements rather than groundbreaking innovations. Let’s explore whether AI is genuinely evolving quickly or if it’s stuck in a cycle of repetition.
Rapid Evolution in AI
1. Breakthrough Technologies:
– Generative AI: Tools like GPT-4 and DALL-E are pushing the boundaries of what AI can create, from generating human-like text to creating images based on textual descriptions.
– Reinforcement Learning: Advances in reinforcement learning are enabling AI systems to solve complex problems by learning from their interactions with the environment, as seen in AI mastering games like Go and StarCraft II.
2. Increased Computational Power:
– Hardware Improvements: The development of more powerful GPUs and specialized AI hardware like TPUs (Tensor Processing Units) is accelerating AI research and deployment.
– Cloud Computing: Scalable cloud computing resources allow researchers and businesses to train large models more efficiently, driving innovation and accessibility.
3. Expansion into New Domains:
– Healthcare: AI is making strides in areas such as drug discovery, diagnostic imaging, and personalized medicine.
– Autonomous Vehicles: AI technologies are continuously evolving to improve the safety and efficiency of self-driving cars.
4. Advances in Natural Language Processing:
– Conversational Agents: AI-powered chatbots and virtual assistants are becoming more sophisticated, understanding and responding to human language with greater accuracy.
– Translation and Language Models: Improvements in translation services and language models are making cross-lingual communication more seamless.
Repetition and Incremental Changes
1. Recycling Old Concepts:
– Algorithms: Many new AI models are built on existing algorithms with minor tweaks. Innovations often involve combining established techniques in novel ways rather than introducing entirely new methodologies.
– Research Papers: There’s a growing concern that the field is producing a large volume of incremental research papers that contribute to marginal improvements rather than significant breakthroughs.
2. Commercialization of Existing Technologies:
– Product Iterations: New AI products often represent iterative improvements of previous models rather than entirely new inventions. For example, new versions of language models or recommendation systems build upon their predecessors.
3. Hype vs. Reality:
– Buzzwords: AI is frequently marketed with buzzwords that promise more than they deliver. Technologies like “AI-powered” solutions often involve applying existing AI techniques to new problems without significant innovation.
4. Ethical and Societal Challenges:
– Bias and Fairness: Despite advancements, AI systems continue to struggle with issues like bias and fairness. Addressing these challenges often involves revisiting and refining existing approaches rather than discovering entirely new solutions.
Balancing Act: Innovation and Iteration
AI’s rapid evolution is evident in many areas, but it’s also clear that some aspects of the field involve repetition and incremental progress. Here’s how the balance is struck:
– Combining Innovation and Iteration: Most significant advancements in AI involve a blend of innovative ideas and iterative improvements. For example, breakthroughs in deep learning often build upon earlier concepts with refinements and new applications.
– Focus Areas: Different sectors within AI may evolve at different rates. While some areas experience rapid innovation, others might see slower, more incremental changes.
Conclusion
The AI field is characterized by a mix of rapid evolution and repetition. While groundbreaking innovations are frequent, many advancements involve refining existing technologies and approaches. Understanding this dynamic is essential for navigating the AI landscape and recognizing both the transformative potential and the incremental nature of current advancements. As AI continues to evolve, the balance between revolutionary breakthroughs and iterative improvements will shape its future trajectory.