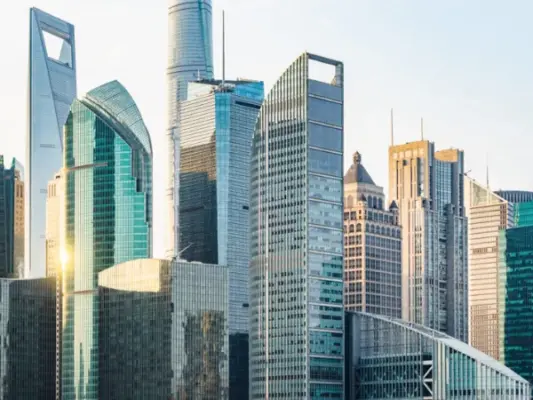
Planning a fun day out with friends can sometimes feel like solving a puzzle with everyone’s different preferences. But fret not! We’re here to make it simpler with the power of AI integrated into Maps. Whether you have a specific spot in mind or just want to explore, our AI-driven feature is your perfect companion. By tapping into Maps’ vast database of over 250 million places and insights from our community of 300 million contributors, we’re revolutionizing the way you discover new places.
This exciting feature is currently in its early access phase for select Local Guides in the U.S., allowing us to fine-tune it based on their feedback. Here’s how it works: Let’s say you’re in San Francisco and in the mood for some vintage shopping. Just ask Maps for “places with a vintage vibe in SF,” and our AI models will analyze nearby businesses, photos, ratings, and reviews to offer you trustworthy recommendations. Results are neatly categorized for easy browsing, complete with photo carousels and review summaries to help you make informed decisions.
But why stop there? If hunger strikes, keep the conversation going with a follow-up question like “How about lunch?” Maps will suggest dining options that match the vintage ambiance you’re craving, such as a cozy diner nearby. You can then save these spots for later or share them with friends.
Our AI-powered suggestions are also perfect for spontaneous plans or unexpected changes. Caught in a sudden downpour? Just ask Maps for “activities for a rainy day” to find indoor options like comedy shows or movie theaters. And if you’re with kids, inquire about “options for kids” to discover family-friendly activities like bowling alleys or children’s museums.
This experimental feature heralds a new era in place discovery, making it easier than ever for you to explore the world. We’re thrilled to continue refining Maps with AI and can’t wait to share this innovative technology with all users in the future.
Navigating the Future of AI in Healthcare Regulation
Artificial intelligence (AI) has the potential to transform healthcare, but concerns about regulatory oversight are on the rise. To address these concerns, the MIT Abdul Latif Jameel Clinic for Machine Learning in Health (Jameel Clinic) recently hosted a thought-provoking conference on AI and Health Regulatory Policy.
Regulators, industry experts, and faculty came together to discuss the challenges and opportunities in regulating AI in healthcare. Key topics included the need for transparency in AI decision-making processes, the impact of AI on drug approval procedures, and the role of medical professionals in shaping regulatory frameworks.
A major point of debate was the FDA’s approval process for AI-driven medical devices. While safety and efficacy evaluations are required, there is ongoing discussion about whether AI algorithms should fully disclose their decision-making mechanisms for approval. This highlights the importance of transparency and accountability in AI regulation.
Participants also stressed the importance of collaboration among stakeholders to address regulatory gaps and ensure patient safety. Medical professionals emphasized the need for consulting clinicians in the development and evaluation of AI technologies to mitigate potential risks.
Additionally, concerns were raised about the availability of high-quality data for training AI models. Researchers emphasized the importance of access to comprehensive datasets for developing effective AI solutions. Efforts to promote data sharing and collaboration among researchers were recognized as crucial steps in advancing AI in healthcare.
Overall, the conference provided a platform for meaningful dialogue and collaboration among stakeholders in the healthcare and AI industries. Moving forward, the Jameel Clinic is committed to fostering open discussions and developing innovative solutions to address the regulatory challenges of AI in health.
Empowering Robots with Multimodal AI for Better Planning
While humans find everyday tasks intuitive, robots require detailed planning and coordination. MIT’s Improbable AI Lab has developed an innovative multimodal framework, Compositional Foundation Models for Hierarchical Planning (HiP), to assist robots in executing complex plans for various tasks.
HiP integrates expertise from three distinct foundation models trained on language, vision, and action data. Unlike previous systems, HiP does not rely on paired data, making it more cost-effective and transparent. By leveraging separate models for different decision-making processes, HiP enhances the robot’s ability to reason and adapt to new information.
The hierarchical planning process of HiP involves pre-training each component on diverse datasets, including internet data beyond robotics. The system begins planning with a large language model (LLM) to outline abstract task plans, which are then refined using a video diffusion model to incorporate environmental information. Finally, an egocentric action model maps out specific actions based on visual observations, enabling the robot to execute tasks effectively.
Evaluation tests have shown HiP’s superior performance in manipulation tasks compared to existing frameworks. The system adapts to changes in the environment and successfully completes complex objectives, demonstrating its potential for real-world applications.
Looking ahead, the Improbable AI Lab aims to further enhance HiP’s capabilities and explore its deployment in various robotics scenarios. By leveraging multimodal AI models, HiP offers a promising solution for enhancing robotic planning and execution in diverse environments.